Rough Paths: gaining insights and building solutions
Researchers in the Mathematical Institute are using the mathematics of ‘rough paths’ and signatures to enhance the analysis of complex streams of data – helping to identify practical solutions to real-world challenges.
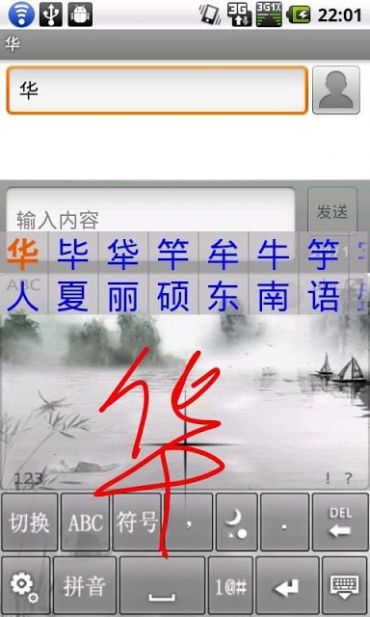
The methodology has been a key contributor to prize winning practical applications ranging from recognition of finger-drawn Chinese characters on mobile devices to analysing health data.
In a paper published in the Annals of Mathematics (2010), Professors Terry Lyons and Ben Hambly showed how ‘mathematical signatures’ from ‘rough path theory’ could be faithfully used to capture the key features of an evolving situation, capturing patterns and allowing accurate prediction and analysis. This lead Lyons and his team to develop signatures into an effective tool to describe the interactions between complex data streams in data science.
Lyons comments: “Multimodal data streams are found in a huge range of situations and on all scales. Many analysis techniques aren’t equipped to deal with them as they often treat each mode independently and can’t deal well with interactions between channels, randomness, or gaps in the data. The rough path model effectively addresses these challenges.”
In 2012, Lyons started to interact with Ben Graham (then at University of Warwick, now at Facebook, and an early expert on deep neural networks). Putting two technologies together (signatures, and deep convolutional neural nets), Ben Graham won the ICDAR 2013 competition for recognising online Chinese characters. Later (2015), Lyons and his team team began to work with Professor Lianwen Jin from the Information Engineering Department at the Southern China University of Technology (SCUT), to use the model to analyse the pen strokes in Chinese characters.
Prof Jin’s research group already had considerable experience with Chinese handwriting recognition and had developed the mobile phone keyboard app ‘gPen’ which translates handwritten characters into text. The use of the rough paths model enabling effective character recognition in real time, and significantly improved the accuracy and speed of the app (as demonstrated in published articles). The model was incorporated into a new version of the software and has now been downloaded by over a million users.
Soon after, a leading company, developer of the popular Chinese ‘Pinyin’ input method editor, acquired access to the technology, releasing it to a wider audience through their market-leading ‘mobile keyboard’ for smartphones. While most people in China prefer to use a digital keyboard, an estimated one hundred million people – many of them elderly and not city-based – still prefer to handwrite. The handwriting interface is therefore an important tool allowing those with less digital confidence to access services and information through the Internet and now has around seventy-five million users a day.
Rough path models have also been used to analyse Intensive Care Unit data to identify those patients most likely to develop sepsis – a rapid onset condition with potentially devastating consequences. The model was the first placed entry (out of more than 100) in the PhysioNet 2019 ‘Early Prediction of Sepsis from Clinical Data’ challenge, whose goal was the early detection of sepsis using physiological data – ideally six hours before the clinical diagnosis.
The signature model has also been used to analyse data to assist in mental health diagnosis, to analyse human movement and strengthen cybersecurity, and has contributed to prize winning work relating to the simulation of financial markets.
Terry Lyons says: “The ability to analyse complex data streams helps us to better understand the world around us, allowing us to identify solutions and take actions to address the challenges we face. I’m delighted to see the mathematical models of rough path theory come of age as powerful applied tools. The progress we make never ceases to surprise me and some of it can be found at our DataSig website.”
Terry Lyons is Wallis Professor of Mathematics Emeritus and Professor
Research team
- Other members of the team in Oxford during development included:
- Ben Hambly, Professor, Lajos Gergely Gyurkó, Post-doctoral Research Assistant, Hao Ni, Post-doctoral Research Assistant, Harald Oberhauser, Post-doctoral Research Assistant and now Associate Professor in Oxford.
- The collaboration with SCUT continues and Weixin Yang, who played a key role in developing the signature version of gPen, is now in Oxford as part of the DATASIG team.
Funders: ERC, Man Group plc, EPSRC, The Alan Turing Institute and particularly their Defence and Security, Data Centric Engineering, and Office of National Statistics Programmes, The Hong Kong Innovation and Technology Commission (CIMDA)
Terry Lyons is very grateful for the funding he has received which enabled this research. In particular, he held an ERC advanced grant (Grant agreement ID: 291244 2012-2017) and was Director of the Oxford Man Institute of Quantitative Finance, a research institute of Oxford University funded by Man Group plc. His research is supported in part by the EPSRC (DataSıg grant number EP/S026347/1), in part by The Alan Turing Institute (under the EPSRC grant EP/N510129/10), the Data Centric Engineering Programme (under the Lloyd’s Register Foundation grant G0095), the Defence and Security Programme (funded by the UK Government) and the Office of National Statistics Programme (funded by the UK Government) and in part by the Hong Kong Innovation and Technology Commission (InnoHK Project CIMDA).