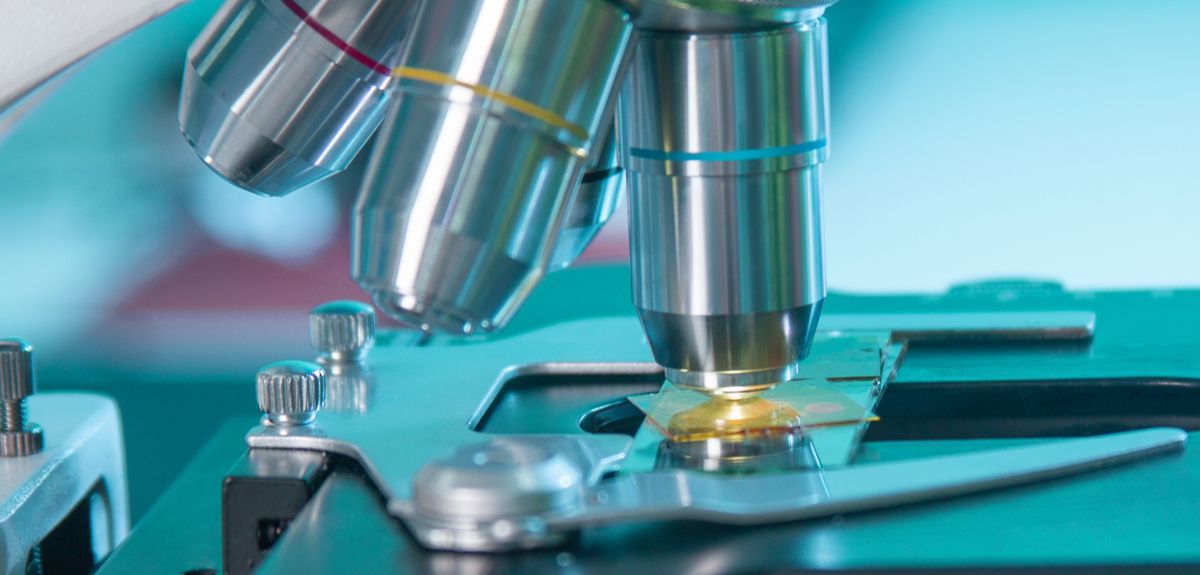
Image credit: Shutterstock
New digital classification method using AI developed for colorectal cancer
A new study from S:CORT demonstrates an easy, cheap way to determine colorectal cancer molecular subtype using AI deep-learning digital pathology technology.
Understanding the molecular subtype of a cancer is becoming an importance part of the diagnostic process as it helps a doctor better understand a patient’s prognosis, determine the best course of action for treatment and helps researchers devise new, more-efficient, precision therapies.
Colorectal cancer (CRC) currently has four known molecular subtypes which are identified on the basis of its RNA expression profile, using RNA analysis. But the process of RNA analysis is costly, technically challenging and it requires a specialist to interpret data to determine the subtype. In order to more efficiently and cheaply determine the molecular subtype of a patient’s CRC, there is a need to use more easily-acquired data on a tumour and categorise its subtype based on an automated technique.
One prevalent source of data in cancer diagnostics is images, such as histological images of the microscopic anatomy of a cancer. Almost all CRC patients already have a sample of their tumour inspected by a pathologist as part of their standard care.
Caption: Image information can be used to predict the molecular classification of patient tumor samples in colour, using state-of-the-art deep learning models in pathology.
A new paper published by Dr Korsuk Sirinukunwattana, Dr Enric Domingo, Prof Viktor H Koelzer & Prof Jens Rittscher from the Stratification in Colorectal Cancer consortium (S:CORT), has demonstrated the potential for using these images to determine molecular subtype.
Through the training of a deep-learning neural network, using over 1,000 tumour samples, the team discovered that AI could be trained to distinguish between the four molecular subtypes using images alone.
Usually, histological images are a modest indicator of how colorectal cancers have progressed, and there is currently no way to determine the molecular subtype of a tumour by the human eye alone, without doing a gene profile on tumour samples. However, this new study demonstrates that technology can be trained to identify the minute morpho-molecular differences between molecular subtypes.
This new concept, called Image-based Consensus Molecular Subtyping (imCMS), will allow images to be interpreted through association of tile-level predictions with morphology, molecular features and outcome data.
Furthermore, this study also demonstrated that AI is able to classify the molecular subtype of samples that were previously unclassifiable by the standard process of RNA expression profiling. It also provides information for the spatial distribution of each subtype within a tumour.
This AI technology will have huge implications on the standardisation of classifying molecular subtypes of CRC. It opens new opportunities to create simple, cheap and reliable ways to quickly stratify a patient’s colorectal cancer, and decreases the demand for specialist input that is not always immediately available.
It has potential to reveal new avenues for the translation of the classification of CRC subtypes into clinical practice and increase availability of molecular stratification in low-resource settings, helping to make accurate cancer diagnoses and appropriate treatment more accessible to everyone.
About S:CORT and the researchers
For more information about the Stratification in Colorectal Cancer consortium (S:CORT) see here.
Samples for this study were collected by the S:CORT program and The Cancer Genome Atlas (TCGA). The S:CORT consortium is a Medical Research Council stratified medicine consortium jointly funded by the MRC and CRUK. This work was further supported by the National Institute for Health Research (NIHR) Oxford Biomedical Research Centre.
This study is a joint venture conceived by Prof Tim Maughan (Dept. of Oncology), Prof Ian Tomlinson (Institute of Genetics and Molecular Medicine, Edinburgh), Prof Jens Rittscher (Institute of Biomedical Engineering) and Prof Viktor H Koelzer (University Hospital and University of Zurich; Honorary Senior Clinical Researcher, Dept. of Oncology, University of Oxford;)
S:CORT consists of a team of clinicians, healthcare professionals, academics and scientists across the UK, lead from Oxford by Prof. Tim Maughan, and seeks to apply cutting edge molecular diagnostic and histopathological technologies to improve and tailor the treatment of colorectal cancer patients.