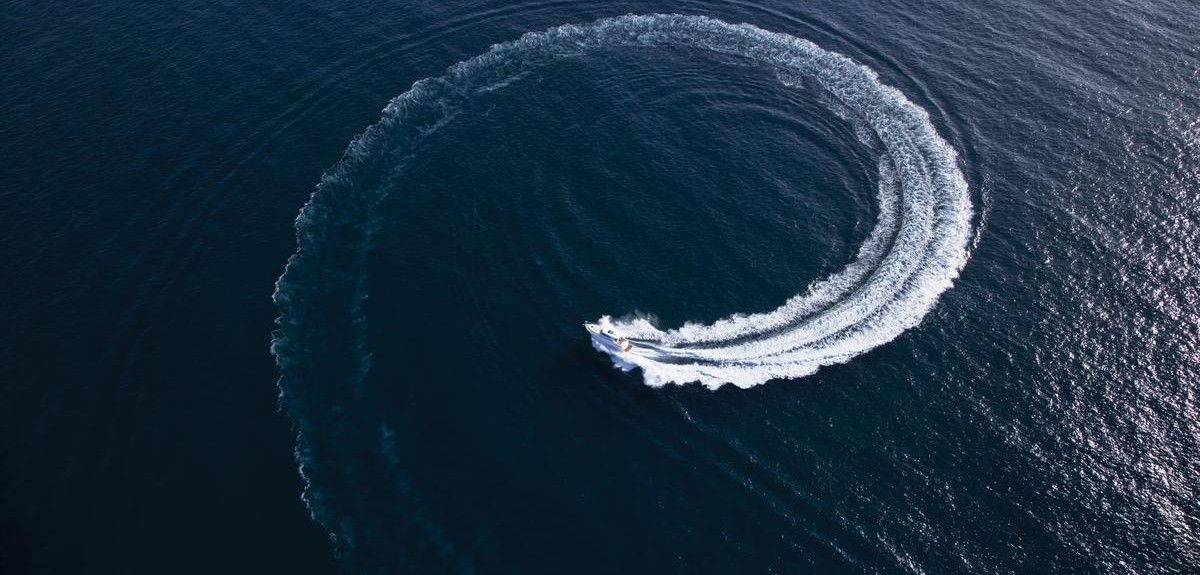
Image credit: EPSTOCK/ Shutterstock
Better engine, worse compass
Researchers have shed light on a critical paradox of modern medical research – why research is getting more expensive even though the cost and speed of carrying out many elements of studies has fallen hugely. In a paper in journal PLOS ONE, they suggest that improvements in research efficiency are being outweighed by accompanying reductions in effectiveness.
Faster, better, cheaper
Modern DNA sequencing is more than a billion times faster than the original 1970s sequencers. Modern chemistry means a single chemist can create around 800 times more molecules to test as possible treatments as they could in the early 1980s. X-ray crystallography takes around a thousandth of the time it did in the mid-1960s. Almost every research technique is faster or cheaper – or faster and cheaper – than ever.
Added to that are techniques unavailable to previous generations of researchers – like transgenic mice, or computer-based virtual models underpinned by processing power that itself gets more powerful and less expensive every year.
Lower costs yet higher budgets?
So why has the cost of getting a single drug to market doubled around every nine years since 1950? To put that into context, for every million pounds spent on drug research in 1950, in 2010, despite all the advances in speed and all the reductions in individual costs, you needed to spend around £128,000,000 to achieve similar success.
Those figures may explain why research in certain areas has stalled. For example, in the forty years between 1930 and 1970, ten classes of antibiotics were introduced. In the forty-five years since 1970, there have been just two.
Better engine, worse compass
This question is tackled by two researchers in a paper published in journal PLOS ONE, including Dr Jack Scannell, an associate of the joint Oxford University-UCL Centre for the Advancement of Sustainable Medical Innovation (CASMI).
Applying a statistical model, they show that what is critical to the overall cost of research is not the brute force efficiency of particular processes but the accuracy of the models being used to assess whether a particular molecule, process or treatment is likely to work.
Dr Scannell explains: 'The issue is how well a model correlates with reality. So if we are looking for a treatment for condition X and we inject molecule A into someone with it and watch to see what happens, that has high correlation in that person – a value of 1. It also has some insurmountable ethical issues, so we use other models. Those include animal testing, experiments on cells in the lab or computer programmes.
A reduction in correlation of 0.1 could offset a ten-fold increase in speed or cost efficiency.
Dr Jack Scannell, Centre for the Advancement of Sustainable Medical Innovation
'None of those will correlate perfectly, but they all tend to be faster and cheaper. Many of these models have been refined to become much faster and cheaper than when initially conceived.
'However, what matters is their predictive value. What we showed was that a reduction in correlation of 0.1 could offset a ten-fold increase in speed or cost efficiency. Let's say we use a model 0.9 correlated with the human outcome and we get 1 useful drug from every 100 compounds tested. In a model with 0.8 correlation, we'd need to test 1000; a 0.7 correlation needs 10,000; a 0.6 correlation needs 100,000 and so on.
'We could compare that to fitting out a speedboat to look for a small island in a big ocean. You spend lots of time tweaking the engine to make the boat faster and faster. But to do that you get a less and less reliable compass. Once you put to sea, you can race around but you'll be racing in the wrong direction more often than not. In the end, a slower boat with a better compass would get there faster.
'In research we've been concentrating on beefing up the engine but neglecting the compass.'
He adds that beyond the straightforward economics of drug discovery, the use of less valid models may also explain why it is getting harder to reproduce published scientific results – the so-called reproducibility crisis. Models with lower predictive values are less likely to reliably generate the same results.
Retiring the super models
There are two possible explanations for this decline in the standard of models.
When the medical problem is solved, the commercial drive is no longer there for drug companies and the scientific-curiosity drive is no longer there for academic researchers.
Dr Jack Scannell, Centre for the Advancement of Sustainable Medical Innovation
Firstly, good models get results. There is no point finding lots of cures for the same disease, so the best models are retired. If you’ve invented the drugs that beat condition X, you don’t need a model to test possible drugs to treat condition X anymore.
Dr Scannell explains: 'An example would be the use of dogs to test stomach acid drugs. That was a model with high correlation and so they found cures for the condition. We now have those drugs. You can buy them over the counter at the chemist, so we don't need the dog model. When the medical problem is solved, the commercial drive is no longer there for drug companies and the scientific-curiosity drive is no longer there for academic researchers.'
The reverse of this is that less accurately predictive models remain in use because they have not yielded a cure, and scientists keep using them for want of anything better.
Secondly, modern models tend to be further removed from living people. Testing chemicals against a single protein in a petri dish can be extremely efficient but, Scannell suggests, the correlation is lower: 'You can screen lots of chemicals at very low cost. However, they fail because their validity is often low. It doesn't matter that you can screen 1,000,000 drug candidates – you've got a huge engine but a terrible compass.'
He cautions that without further research it is not clear how much the issue is caused by having 'used up' the best predictive models or how much it is a case of choosing to use poor predictive models.
Re-aligning the compass
Is it not peculiar that the first useful antibiotic, the sulphanilamide drug prontosil was discovered by Gerhard Domagk in the 1930s from a small screen of available [compounds] (probably no more than several hundred), whereas screens of the current libraries, which include ~10,000,000 compounds overall, have produced nothing at all?
At one level, it is easy to see why efficiency has been prioritised over validity. Activity is easy to measure, simple to report and seductive to present. It is easy to show that you have doubled the number of compounds tested and it sounds like that will get results faster.
But Dr Scannell and his co-author Dr Jim Bosley say that instead we should invest in improving the models we use. They highlight the focus on model quality in environmental science, where the controversy around anthropomorphic global warning has pushed scientists to explain and justify the models they use. They suggest that this approach – known as 'data pedigree' – could be applied in health research as well, suggesting that it should be part of grant-makers' decision processes. That would ensure that researchers would try to fine-tune their compass as much as their engine.
Dr Scannell concludes: 'If we are to harness the power of the incredible efficiency improvements we have seen, we must also be better at directing all that brute-force power. Better processes must be allied to better models in order to generate better results. We are living with the alternative – a high-speed ride that, all too often, goes nowhere fast.'
The paper, When Quality Beats Quantity: Decision Theory, Drug Discovery, and the Reproducibility Crisis is published in PLOS ONE (DOI: 10.1371/journal.pone.0147215)