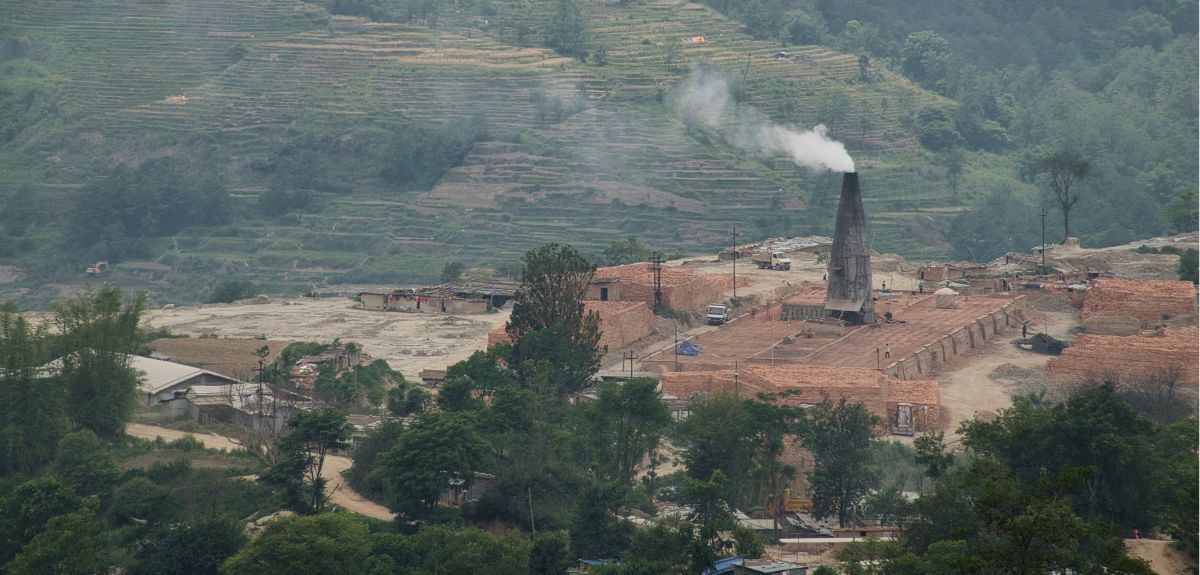
AI could help detect unregulated sources of air pollution in South Asia, new project shows
A collaboration between the University of Oxford and Lahore University of Management Sciences has combined the power of artificial intelligence with remote satellite imagery to help tackle the problem of unregulated brick kilns across South Asia. These kilns are a major source of air pollution, a key contributor to climate change, and notorious for people trafficking and modern-day slavery.
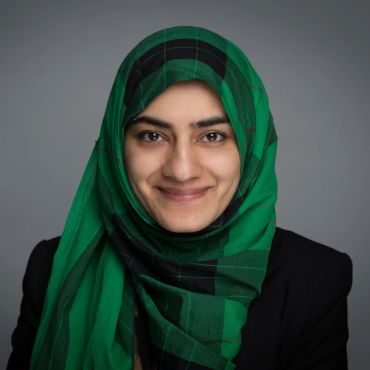
With the Brick Kiln Belt spanning 1.5 million km2 and crossing country borders, it would be impossible for law enforcers in these resource-poor areas to monitor it using ‘on the ground’ methods alone. An alternative approach is to identify the distinct shape of brick kilns from aerial images captured by satellites. Doing this manually, however, is highly time consuming and prone to error. Although some studies have applied machine learning to aerial images to try to detect illegal brick kilns automatically, none have so far produced a highly scalable solution.
A collaboration between the Center of Urban Informatics, Technology and Policy at Lahore University of Management Sciences, Pakistan, and the University of Oxford’s Centre for Statistics in Medicine, developed an improved model that can detect brick kilns from aerial images up to 21 times faster than existing techniques. The model uses a unique two-stage computational process where images are first filtered at a low-resolution so that only those that contain a likely brick kiln proceed to the next stage. This lowers the computational power required, making it a highly scalable solution.
The exact numbering and location of kilns is needed to understand and address the brick kiln sector’s climate, environmental and health impacts. The model provides a rapid, accurate and highly scalable tool to automatically detect and map brick kilns across this vast region.
Associate Professor Sara Khalid, Centre for Statistics in Medicine, University of Oxford.
‘This proposed model overcomes what has been a severe limitation with using machine learning approaches for brick kiln detection’ said Associate Professor Sara Khalid, the Oxford lead for the study. ‘Models based on low-resolution images are rapid but do not meet the required accuracy. On the other hand, models that use high-resolution images are more accurate but require lengthy processing and unfeasible amounts of computational power.’
‘In the first stage of our model, the images are first filtered at low-resolution by an algorithm that identifies potential brick kilns based on their spectral properties’ added co-investigator Dr Usman Nazir (Lahore University of Management Sciences). ‘This means that only a small subset proceeds to the second stage of fine-grained localisation, where objects are detected at high-resolution using a deep learning-based model. Having these two stages reduces the required computational power considerably, speeding up the process.’
The team trained the model using a dataset of 1300 aerial images, each with a box manually drawn around a brick kiln. This enabled the model to ‘learn’ the typical features of a brick kiln, including the typical dimensions, orientation, and surrounding vegetation. The research team then tested it on publicly available aerial maps covering three cities: Deh Sabz (Afghanistan), New Delhi (India), and Kasur (Pakistan).
This proposed model fuses spectral analysis with deep learning to boost performance and reduce computational time. Another novelty is that this model works effectively on freely available public data, overcoming the constraint to rely on high-resolution imagery which is not often publicly available.
Associate Professor Murtaza Taj, Center of Urban Informatics, Technology and Policy, Lahore University of Management Sciences.
Compared with existing machine learning-based approaches, the two-stage model was significantly faster whilst maintaining a similar level of accuracy. ‘Previous models took on average 674 seconds to process the three maps whilst our proposed two-stage approach had a compute time of only 38 seconds’ said co-investigator Associate Professor Murtaza Taj (Lahore University of Management Sciences). When scaled up to the entire Brick Kiln Belt, previous models would take between 92 days and 5 years to process all the data, whilst the new model would only take 4 days.
In the near future, the research team intend to collaborate with governments of Brick Kiln Belt countries, so that the model can be used by policy makers to detect illegal kiln activity. This is particularly important during the winter smog period, when brick kiln workers are exposed to unsafe concentrations of particulate matter, resulting in high incidences of respiratory diseases such as lung cancer, chronic bronchitis, and asthma.
Associate Professor Khalid added that the model could have many uses beyond geo-localisation of brick kilns. ‘The multi-stage strategy could easily be adapted to detect other objects that have distinct spectral signatures and well-defined shapes, such as industrial units, oil tanks, and warehouses, or to quantify urban and natural cover. This opens up many possible uses in law enforcement, spatial planning, and climate change mitigation policy.’
The project draws on Associate Professor Khalid’s expertise in applying artificial intelligence to international real-world data. In 2021, she set up the Planetary Health Informatics Group at the University of Oxford to develop digital platforms and open science tools to address health, environment, and climate challenges.
‘This project really aligns with my motivation to see artificial intelligence applied responsibly to solve both human and planetary health problems’ Associate Professor Khalid concluded. ‘Importantly, such tools can empower local governments and communities to monitor levels of pollution from unregulated industries themselves and act against illegal activity.’
The study ‘Mitigating climate and health impact of small-scale kiln industry using multi-spectral classifier and deep learning’ has been published on arXiv.
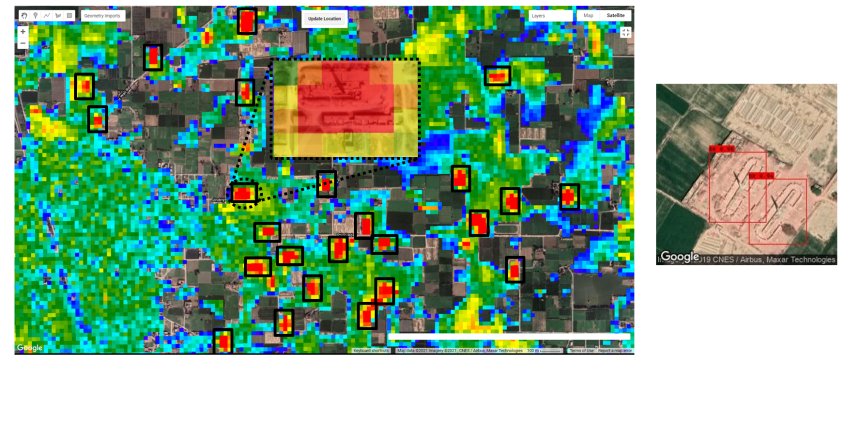