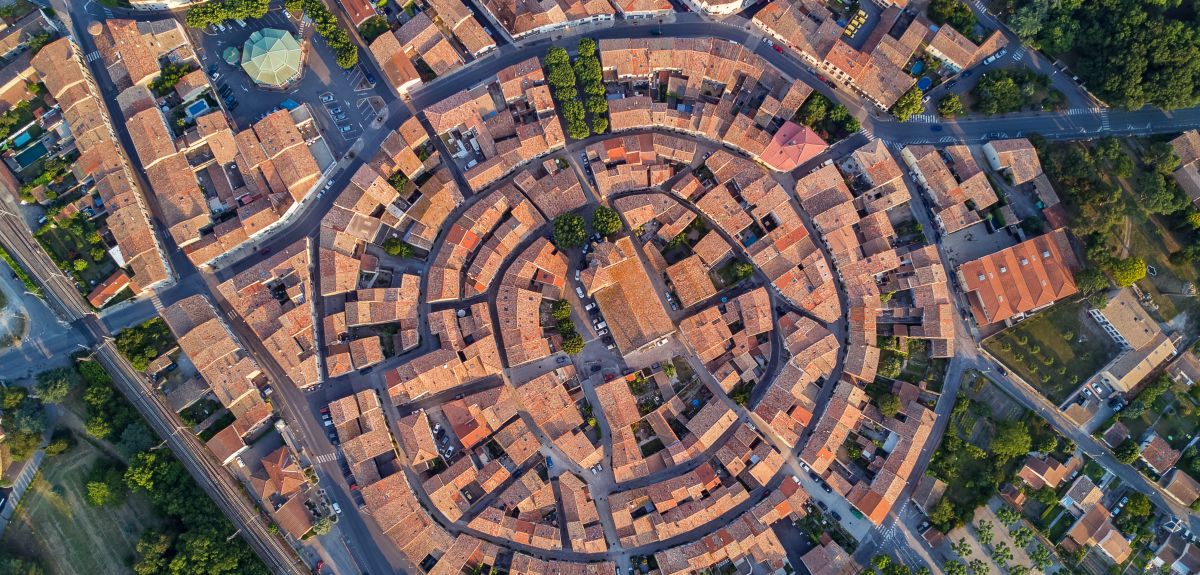
Credit: Shutterstock
Research emphasises need for COVID-19 vigilance in tight-knit communities
Small, close-knit communities are at high risk for rapid, intense COVID outbreaks especially if they haven’t yet experienced outbreaks of COVID-19, shows a new study by the University of Oxford and Northeastern University, Boston.
The paper, published today in the journal Nature Medicine, shows that whether COVID outbreaks come to a fast, dramatic peak or whether they are long and drawn-out is more closely related to city layout and social structures than city size and density. Traditional epidemic models are based on a single population, but in reality, large cities are made up of many linked communities. This enables an outbreak to travel through city populations community-by-community resulting in a prolonged, sustained epidemic.
Places with higher population densities, single city-centres and business districts, and large numbers of connections between different communities are likely to suffer larger epidemics. This could go some way to illustrating the mechanism why the outbreaks in large cities like NYC, London and Madrid had much higher case numbers compared to more rural areas. And potentially explain why university halls of residence have proved to be so vulnerable to rapid outbreaks.
Dr Moritz Kraemer, Associate of the Oxford Martin Programme on Pandemic Genomics and Branco Weiss Fellow at Oxford University’s Department of Zoology, said: 'Public health measures are often focused on “flattening the curve” but this research shows that what that curve looks like will be very different from city to city, town to town, or even neighbourhood to neighbourhood. Measures should be developed with that perspective in mind, for example thinking about how an intervention reduces the circle of contacts of an average individual in each location.
If a disease keeps reseeding itself in communities that are not in the midst of an outbreak, it will perpetuate the wider epidemic
‘Our research also shows that the traditional wisdom in public health, that once local transmission has started then new importations of a disease from outside are no longer a priority to control, needs to be re-assessed. If a disease keeps reseeding itself in communities that are not in the midst of an outbreak, it will perpetuate the wider epidemic.’
The paper includes a map of risk intensity covering 310 cities across the world showing those at risk of shorter, more overwhelming peaks, such as Novosibirsk, Russia and Ulaanbaatar, Mongolia, and those likely to have more extended outbreaks, including Madrid, Spain and Colombo, Sri Lanka.
This paper’s hypothesis, that a hitherto unaffected area given a single introduction of COVID-19 can lead to a rapid peak in cases when individuals had high rates of contact within social units, such as households, care homes and prisons, explains that outbreak pattern
Professor Samuel Scarpino of the Emergent Epidemics Lab, Northeastern University, Boston, said: ‘This data-led research provides a clear explanation for some of the key anecdotes of the COVID-19 pandemic. For example, a rural wedding in Maine has been linked to 170 cases and seven deaths. This paper’s hypothesis, that a hitherto unaffected area given a single introduction of COVID-19 can lead to a rapid peak in cases when individuals had high rates of contact within social units, such as households, care homes and prisons, explains that outbreak pattern.
‘Smaller, highly connected communities that haven’t experienced a COVID-19 case should therefore be more vigilant on adhering to public health measures, not less.’
Smaller, highly connected communities that haven’t experienced a COVID-19 case should therefore be more vigilant on adhering to public health measures, not less
The paper, ‘Crowding and the shape of COVID-19 epidemics’ used highly spatially resolved daily epidemiological data from Chinese cities and Italian provinces, climate and population data, and the response to local interventions as measured by human mobility data from Baidu Inc and COVID-19 Aggregated Mobility Research Dataset from earlier this year. This was used to identify drivers of transmission and create a model to predict the risk of high-peak outbreaks based on similar data from 310 cities around the world.
Read the full paper in Nature Medicine: https://www.nature.com/articles/s41591-020-1104-0.