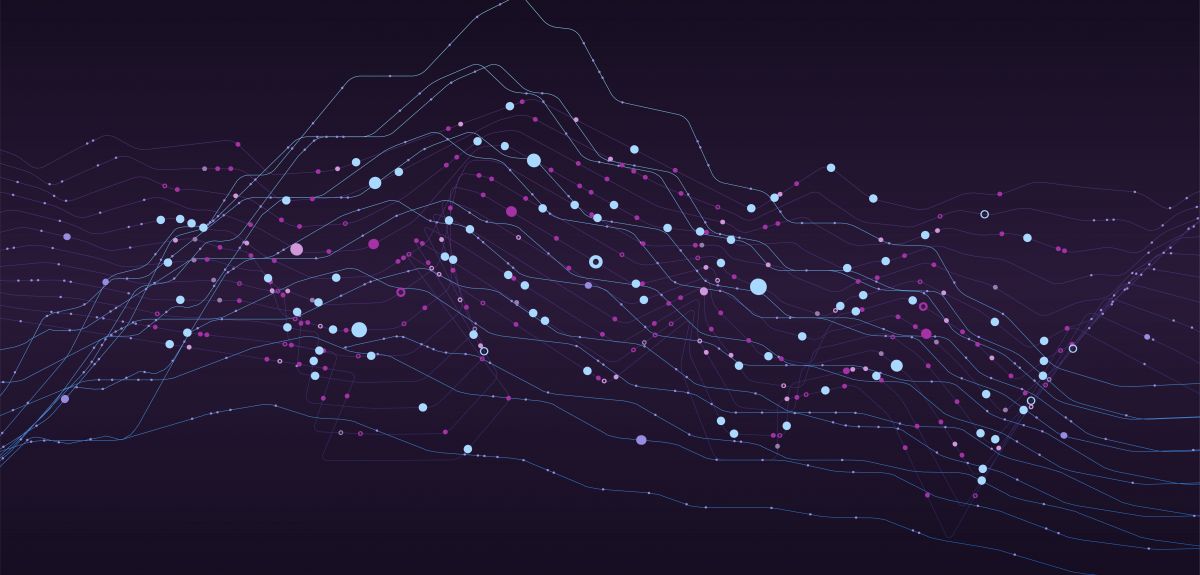
Credit: Shutterstock
Machine learning helps open up new possibilities for quantum devices
Scientists from the University of Oxford, in collaboration with University of Basel and Lancaster University, have developed an algorithm that can be used to measure quantum dots automatically.
The electron spin of individual electrons in quantum dots could serve as the smallest information unit of a quantum computer. Writing in npj Quantum Information, the scientists describe how they can massively speed up this hugely time-consuming process with the help of machine learning.
Their approach to the automatic measurement and control of qubits represents a key step toward their large-scale application.
Dr. Natalia Ares from the University of Oxford’s Department of Materials, said: ‘For the first time, we’ve applied machine learning to perform efficient measurements in gallium arsenide quantum dots, thereby allowing for the characterization of large arrays of quantum devices.’
Professor Dr. Dominik Zumbühl from the University of Basel, said: ‘The next step at our laboratory is now to apply the software to semiconductor quantum dots made of other materials that are better suited to the development of a quantum computer.
‘With this work, we’ve made a key contribution that will pave the way for large-scale qubit architectures.’
For several years, the electron spin of individual electrons in a quantum dot has been identified as an ideal candidate for the smallest information unit in a quantum computer, otherwise known as a qubit.
In quantum dots made of layered semiconductor materials, individual electrons are caught in a trap, so to speak. Their spins can be determined reliably and switched quickly, with researchers keeping the electrons under control by applying voltages to the various nanostructures within the trap. Among other things, this allows them to control how many electrons enter the quantum dot from a reservoir via tunnelling effects. Here, even small changes in voltage have a considerable influence on the electrons.
For each quantum dot, the applied voltages must be tuned carefully in order to achieve the optimum conditions. When several quantum dots are combined to scale in the device up to a large number of qubits, this tuning process becomes enormously time-consuming because the semiconductor quantum dots are not completely identical and must each be characterized individually.
This break-through algorithm will help to automate the process. The scientists’ machine-learning approach reduces the measuring time and the number of measurements in comparison with conventional data acquisition.
The scientists have trained the machine with data on the current flowing through the quantum dot at different voltages. Like facial recognition technology, the software gradually learns where further measurements are needed, with a view to achieving the maximum information gain. The system then performs these measurements and repeats the process until effective characterization is achieved according to predefined criteria and the quantum dot can be used as a qubit.
Read the full article: 'Efficiently measuring a quantum device using machine learning' in npj Quantum Information.